Machine ethics – a question of development
For decades, we have been able to admire the technical possibilities of artificial intelligence at research institutes and industrial trade fairs: recognising people on photos, understanding natural language and situations, conducting dialogues, navigation, driving vehicles, climbing stairs and dancing. Well, not every machine can do all of this, but with enough budget it is conceivable to build an autonomous robot that combines all these capabilities. Autonomous means that the robot makes decisions independently, without a driver or remote control.
However, we still don’t see any general purpose robots rolling along the road. Artificial intelligence has not yet arrived in our everyday lives for a good reason. Apart from the fact that hardly any of us can afford such a household helper financially, there is still a lack of legal questions at the moment. An operating permit for a potentially dangerous machine that moves unattended in public space is likely to be difficult to obtain. However, I have already encountered an autonomous robot on a university corridor, which I took with me in an elevator. (It was too small to press the elevator button itself.) Robots and autopilots have also long been in use in factory halls, in the cockpit of aircraft, in fields or on the rails of the subway, i.e. on a separate private area. The first person killed by a robot was a maintenance mechanic, who unfortunately made a mistake when he had to switch the robot into maintenance mode. This death was therefore not the starting signal for the robots to take over world domination, but an industrial accident, which can happen only too easily even with a shredding machine that is not at all intelligent. But of course such deadly misunderstandings should not affect innocent passers-by.
Rules for ethically correct conduct
Before one begins to make highly philosophical thoughts about machine ethics, one should bear in mind that the question of ethically correct behavior has practically been answered for a long time. A robot should, of course, behave exactly as we would correctly expect a human being to behave in the same situation. If the traffic light shows “red”, the autonomous car should stop, if it is “green” it should drive. Unless there are concrete reasons for an exception, e.g. if a pedestrian walks on the road in spite of red. In decades or even centuries of laborious work, laws, regulations, standards, manuals and teaching materials have been developed for all situations in life. These also apply to robots when they take over our work. In addition, there are often unwritten laws and best practices.
Anyone who deals with ethics knows that adherence to rules and ethics are not congruent in a few individual cases. Many sets of rules do not cover all special cases that can be circumvented in practice by tricks. As soon as ethics is to be incorporated into the machine, such special cases have to be codified.
From a technical point of view, the question is how to teach such rules to the machine. They can be programmed as fixed rules and algorithms. There are already enough known possibilities for it, for example decision trees or state machines. The idea of using neural networks to learn unwritten rules, special cases and subtle differences that are not codified anywhere also seems charming. Like a trainee, artificial intelligence learns implicit knowledge through constant contact with the trainer, his role model and through feedback. From a legal point of view, a possible liability claim in case of robot errors passes from the programmer of the algorithms to the trainer, who may be the owner. This has an advantage for the manufacturer. If, however, one takes into account the fact that artificial intelligence has so far regularly embarrassed itself by learning the wrong connections or adopting the unethical bad habits of its trainers, self-learning no longer seems so attractive. In any case, this approach requires a high-quality learning environment and a trained trainer. That would be a new profession – robot trainer instead of dog trainer?
Software errors are unethical
Suppose we program an artificial intelligence that controls a potentially dangerous machine like a car or other road vehicle, a delivery drone (that could fall on a passer-by’s head) or a care robot (that could overlook a hazard or hurt the patient). For programming, we use laws, textbooks, and instructor testimonials. Is the machine therefore already ethically correct?
Probably. But only if it actually does what it is supposed to do. Error-free software development and thorough testing are required, but unfortunately not perfectly possible. There are rules, standards, and best practices that do not completely prevent errors, but can reduce the risk of undiscovered errors to an ethically and legally acceptable level. Safety-critical systems have already been successfully developed to date. In 2017, for example, not a single passenger plane crashed. In addition to strict standards for all individual parts of the system and for the entire development process, regular, close-meshed quality controls and stringent implementation of the principle of continuous process improvement also contribute to this. Continuous process improvement means an attitude of mind that one can and wants to constantly improve. If you set yourself the goal of making the machine completely error-free, there must be no tolerance for errors. Not only every accident, but also every near-accident and every irregularity must be taken seriously and investigated: How did this come about? What is the cause? How can we prevent a repetition in the future? Where do we need to improve?
Such an uncompromising quality orientation must inevitably lead to an improvement. Unfortunately, it causes costs for analyses, quality assurance measures and changes in the work process. From a business point of view, it is probably also unethical to waste money on unnecessary safety measures. In the case of mass-produced products – which robots and artificial intelligence are not yet – the per-unit price of quality assurance is reduced by the allocation to the mass produced. However, it would still be unethical to cut back on safety and release life-threatening devices onto humanity.
Not everything may be handed over to the machines
So the machines will probably continue to control only those processes that do not take place in public or cannot cause any physical damage. Big data analyses, for example, are concerned with the innocent general public, but rarely endanger human existence. From an ethical and legal point of view, there is still a great hurdle to overcome before machines, software or artificial intelligence can take responsibility for human lives, and in my opinion they should never do so at all.
This would not only be risky, but also a development that cannot be easily reversed. If, for example, in 30 years’ time buses and cars will only be driven by software and drivers and passengers will no longer need a driving licence, they will no longer take a driving test. Driving schools are closing down and traffic rules are being forgotten. The failure of the driving automat (e.g. due to a software error in the latest update) would have more serious consequences than the failure of a traffic light today. Traffic would collapse. The same applies to machine-controlled manufacturing processes, automated management decisions and so on. No one would know exactly what was happening and what was supposed to happen. If something goes wrong here, then it really goes wrong.
The three questions of machine ethics
Machine ethics is thus divided into three different questions:
- How does a machine learn ethical behavior?
- How do we develop error-free autonomous machines, since errors are unethical?
- What responsibility can we ethically leave to the machines? Where can we use them, what decisions can they make themselves?
Of these three questions, I consider the last to be the most important. If we make mistakes, it may not be possible to correct them.
Notes:
Here in our t2informatik Blog you can find more articles from her, including
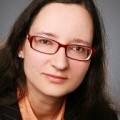
Dr Andrea Herrmann
Dr Andrea Herrmann has been a freelance trainer and consultant for software engineering since 2012. She has more than 28 years of professional experience in practice and research.
Dr Herrmann was most recently a deputy professor at Dortmund University of Applied Sciences and Arts, she has published more than 100 specialist publications and regularly gives conference presentations. She is an official supporter of the IREB Board and co-author of the IREB syllabus and handbook for the CPRE Advanced Level Certification in Requirements Management.